
The Five Most Common Pricing Strategies
A pricing strategy is the method of placing value on a product or service and is the result of a complex set of calculations, research and understanding, and risk-taking ability. It takes into account amongst others segments, market conditions, competitors, trade margins, input costs, consumers’ ability to pay, production and distribution costs, and variable costs. It targets defined customers and competitors.
These strategies can be useful for several reasons, although those reasons may vary from company to company. Choosing the right price for a product allows you to increase the profit margin. Contrary to popular belief, pricing strategies are not always about profit. For example, you may choose to maintain your grip on market share and determine the best or lowest price for a service to prevent competitors from occupying your territory. In these cases, you may be willing to sacrifice profits to focus on competitive prices. But you have to be careful when engaging in such activity. While this can be good for your short term sales, it can also weaken your company.
Determining how much to charge for your product requires more consideration than just calculating your costs and adding a markup. Setting the price too high and you will lose valuable sales. Setting them too low and you will lose valuable revenues. Thankfully, there are dozens of pricing models and strategies that can help you better understand how to set the right price for your audience and revenue goals.
“How much the customer is willing to pay for the product has very little to do with cost and has very much to do with how much they value the product or service they’re buying,” says Eric Dolansky, Associate Professor of Marketing at Brock University in St. Catharines, Ont.
1. Competitor-based Pricing
Competitor-based pricing, also known as competitive pricing or competition-based pricing, is more like plagiarism. For instance, if you decide not to do your homework, then you copy that from those who have already done some. This method looks at the prices set by other businesses in the same area, and then receives those numbers, with a certain percentage plus or minus depending on how your product looks like that day. The dartboard is smaller, as there is more data here, allowing you to trust your competitors to work for you (as long as you trust that they really know what is going on in the market). With competition-based pricing, you can place your products a little below your competition, similar to your competition, or slightly above your competition. If you sell a product and your competitors sell the same product between $20 to $30, you can choose the price between those two numbers. Whatever price you choose, competitive pricing is a way to stay on top of the competition and keep your prices dynamic.
2. Value-based Pricing
A value-based pricing strategy works by determining the true customer’s willingness to pay for a particular product or service using customer data. Most common pricing strategies and methodology do not care about the customer, instead, they focus on internal factors and/or competitive scales to validate prices. However, customers do not care how much it costs you or your competitors, they care how much value they get at a certain price. By focusing on customers, value-based pricing can provide real data, helps you develop high-quality products, and also improves customer loyalty. On the other hand, for this strategy, you need to have constant contact with your different customer profiles because the willingness to pay differs for different customer personas, regions, and even offers. Of course, value-based pricing is not perfect and 100% accurate, but we can get pretty damn close.
3. Cost Plus Pricing
Cost Plus Pricing, also known as markup pricing, is the easiest strategy for estimating prices because businesses that use this strategy, “mark-up” their products depend on how much profit they want to make. In practice, most companies use this method by calculating the cost of production and determine the profit margin they want. To use this strategy, add a limited percentage to your product production costs. For example, suppose you are selling watches. It costs $20 to make watches and you want to make a profit of $20 per sale. You set a value of $40, which is a 100% markup. Cost-plus pricing is very useful when you don’t have complete knowledge about a customer’s budget and there aren’t direct competitors in the market. This type of pricing is generally used by retailers or wholesalers who sell physical products. Overall, Cost-plus pricing is not ideal for big businesses. Despite its simplicity and convenience, this strategy is not the best fit for service-based or SaaS companies, because their products typically offer far greater value than the cost to create them.
4. Dynamic Pricing
The dynamic pricing model is the latest pricing trend of selling the same product to different groups at different prices. For example, global e-commerce giant Amazon is one of the largest retailers using dynamic pricing and update prices every 10 minutes. This is a simple pricing strategy where prices fluctuate depending on the market and customer demand. Hotels, airlines, and utility companies use dynamic pricing by implementing algorithms that consider competitive pricing, demand, and other factors. These algorithms allow companies to change prices depending on when and what the customer is willing to pay for the purchase. Companies know that not all of their customers are the same, so they may be able to get more revenue from some than others. This strategy is also known as surge price, demand price, or time-based price.

5. Key-value item Pricing
Key-value items (KVIs) are the highest profit-making products that contribute significantly to the overall sales of the business. Most of the companies have one or more products that make up the company’s highest sales. For example, a company like Apple has an iPhone as a key-value item that produces a significant portion of the company’s profits.
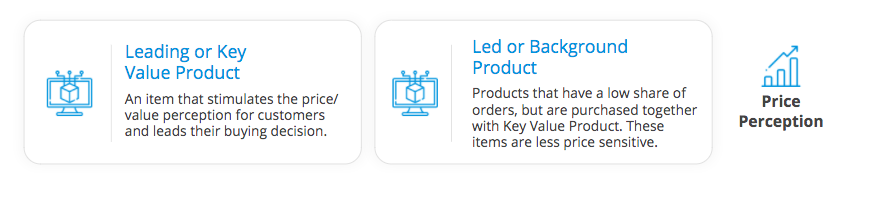
Proper and well organized KVIs and KVCs (Key-Value Categories) lead to balanced pricing decisions along with many other key factors, thereby increasing customer engagement, loyalty, and profit. This allows you to maintain your sales targets such as turnover or margins and achieve their balance well. Finding true KVIs is a non-trivial task.
To find true KVI products, you need to understand the structure of the product basket and correctly assign categories to other products that require different repricing strategies. Before doing anything, companies should list KVCs or the categories that are most attractive to customers and the products in these categories that drive the most KVIs or perceived value and customize them by price area or geography. They, then determine the prices of selected products, including competitors’ prices, current targets, target market share, and profit margin, and price resilience.
In addition to price, KVC and KVI are differentiated from other products in terms of space allocation and layout of the product set, marketing, and promo activity. To optimize pricing strategy, companies should refresh their KVCs and KVIs as often as possible.
With SYMSON you are able to detect and optimize the prices for your Key Value- and Non Key Value Items. With our service we help you map out the Key Value Items and implement a profitable data-driven pricing strategy based on this.
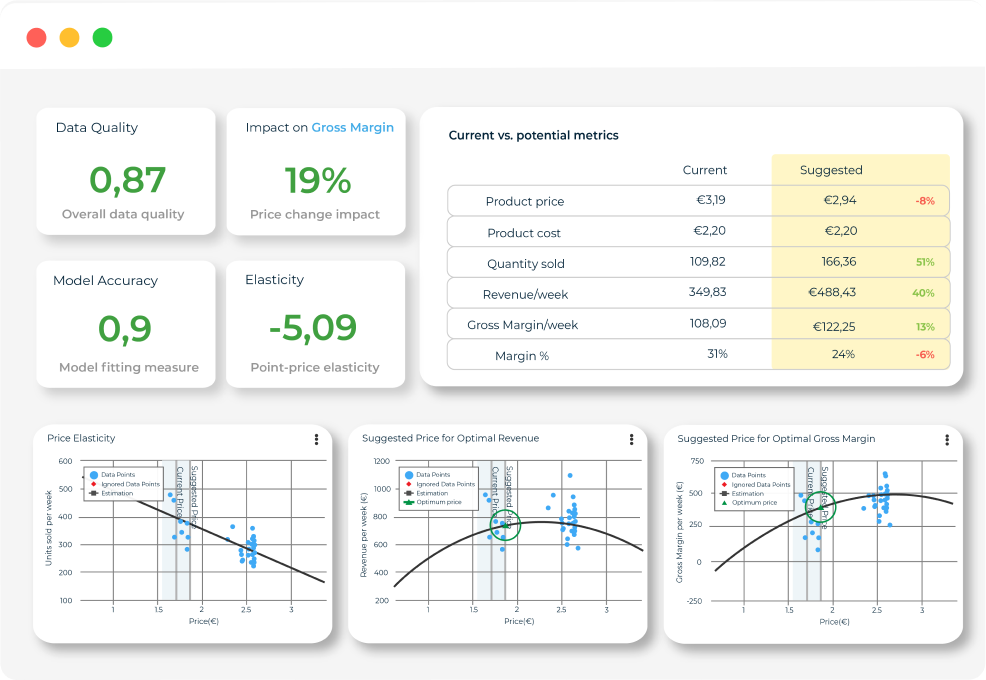
Summary
Pricing is an iterative process and can be daunting for a business that does not have a system fully dedicated to this process. Price is a black box that shines only through a process. Take this knowledge, apply what is useful, and optimize your prices to the point where you can extract maximum cash revenue from the table. And If you do not, then you’ll be losing a large amount of money which can hurt your business in this ever-changing market.
Do you want a free demo to try how SYMSON can help your business with margin improvement or pricing management? Do you want to learn more? Schedule a call with a consultant and book a 20 minute brainstorm session!