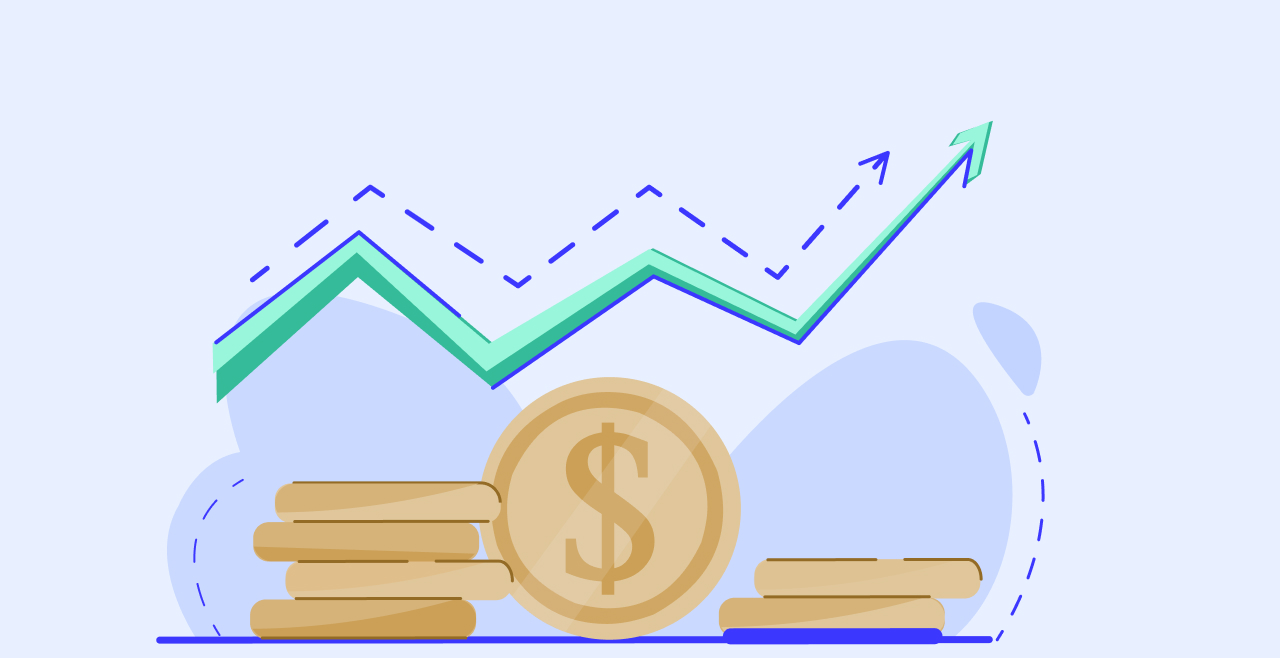
Introduction
We are in an age of unprecedented technological progress. This, combined with the fact that we are just emerging from an unexpected economic recession, is evidence that businesses must evaluate what it looks like to have sustainable growth and success. In this realm, price optimisation is one paramount area.
Recent years have brought forth significant data challenges to make the price elasticity model accurate. This article provides a unique approach to combining human expertise and machine learning to assess products on price sensitivity to give companies new insights and actions on how to improve profitability.
A B2B distribution company we know made an attempt to optimise its margins. The software system they use helps the company to reset price targets in real-time at a product level based upon cost-, stock- and margin levels, but the company desires to move towards a more value-driven approach. Therefore, the management also studies price elasticity to identify and segregate products that are sensitive and insensitive to demand. Management notices that historical data is less relevant to measure price elasticity. Cost price changes mainly drove the past 12 months' price changes. Measuring these as new price points is statistically not correct.
The management ran into a few issues with price elasticity:
- historical data was not clean for optimisation;
- no competitive data was available.
- there are more factors that drive the demand for the product than just the price;
- the sales teams didn’t trust the pricing recommendations features and so wouldn’t use them.
This situation is all too familiar to many companies. Therefore, new optimisation methods have to be developed that take more factors into account and are easier to adopt by sales teams.
Companies require a system that ensures precise and accurate pricing strategies based on the relevant macro to micro parameters and advanced datasets to brace for and combat such downturns. A system that frees up significant time for human judgement towards thoughtful decisions instead of mundane and repetitive ones. In short, the pricing decisions in the future call for the synergy between Machine Learning and human expertise to ensure profitable and sustainable business growth.
Price Optimisation and its Potential
A McKinsey & Company study reports that a 1% rise in price, with stable sales volume, led to an 8% rise in operating profits. More and more intelligent companies are using scientific algorithms and analytics to find optimal prices. This process involves accounting for competitors and price sensitivity. When using these scientific algorithms, it’s crucial to include multiple factors and use platforms and systems which allow them to change prices dynamically in real time.
Case in point: Amazon "reprices millions of items as frequently as every few minutes". Furthermore, according to a study by Forrester Consulting, enterprises using data management systems are 58% more likely to hit their revenue goals and 162% more likely to exceed them. Price optimisation allows companies to transform this data into actionable insights, driving smarter pricing decisions.
Likewise, during uncertain times too, companies can capitalise on such power of technology to enhance profitability, cater to customer needs, and gain a competitive edge- all in a sustainable way.
Why Traditional Pricing Methods Don’t Cut it
The COVID-19 pandemic has been responsible for major changes in consumer behaviour and purchasing patterns. McKinsey and Company report an overall trend in consumers becoming more mindful and spending less on expensive products.
According to a McKinsey & Company report, in the UK alone, 44% of respondents became more mindful of where they spent their money, and 32% shifted to less expensive products to save money.
This along with the disruption of e-commerce and the shift to digital methods has led to the need for more dynamic pricing. Deloitte highlights that FMCG companies sitting "on a treasure-trove of data [ . . . ] can highly benefit from using AI-driven dynamic (portfolio) price setting…”
"The pandemic essentially squeezed ten years of digital sales penetration into three months." - McKinsey & Company.
We also see that large volumes of historical data pre-COVID-19 have been rendered unusable and/or unreliable due to the marked changes seen in the market during and post COVID19.
Falling back on the traditional or manual ways to make those adjustments breeds inaccuracy due to human errors. The procedure currently conjures time and energy and needs to be updated. Therefore, traditional price management methods, based on historical data and fixed pricing models, often fall short in adapting to these rapid changes. From the above data, we can deduce that those enterprises slow to adjust to the crisis with new services, offers and customer-specific pricing strategies had to suffer the blow.
Multiple factors can lead us to label items/services as price sensitive including continuous shifts in buyer purchase behaviour towards specific products, depending on seasonality, current economic health, region, and other aspects alike.
With time and new technological advancements, consumer sensitivity to product prices shifts. Hence, with such changes, it is imperative to research new probable drivers that trigger price sensitivity and experiment to fine-tune the strategy. Likewise, such a step is vital to maintain revenue flow and healthy profit margins regardless of new advancements with time.
At SYMSON, we saw the accuracy of our current price elasticity models decrease, and we saw the need to develop a new method. In response, we developed a 5-step approach to test a new aspect of our pricing strategies.
5-Step Approach to Assess the Price Sensitivity of Your Products
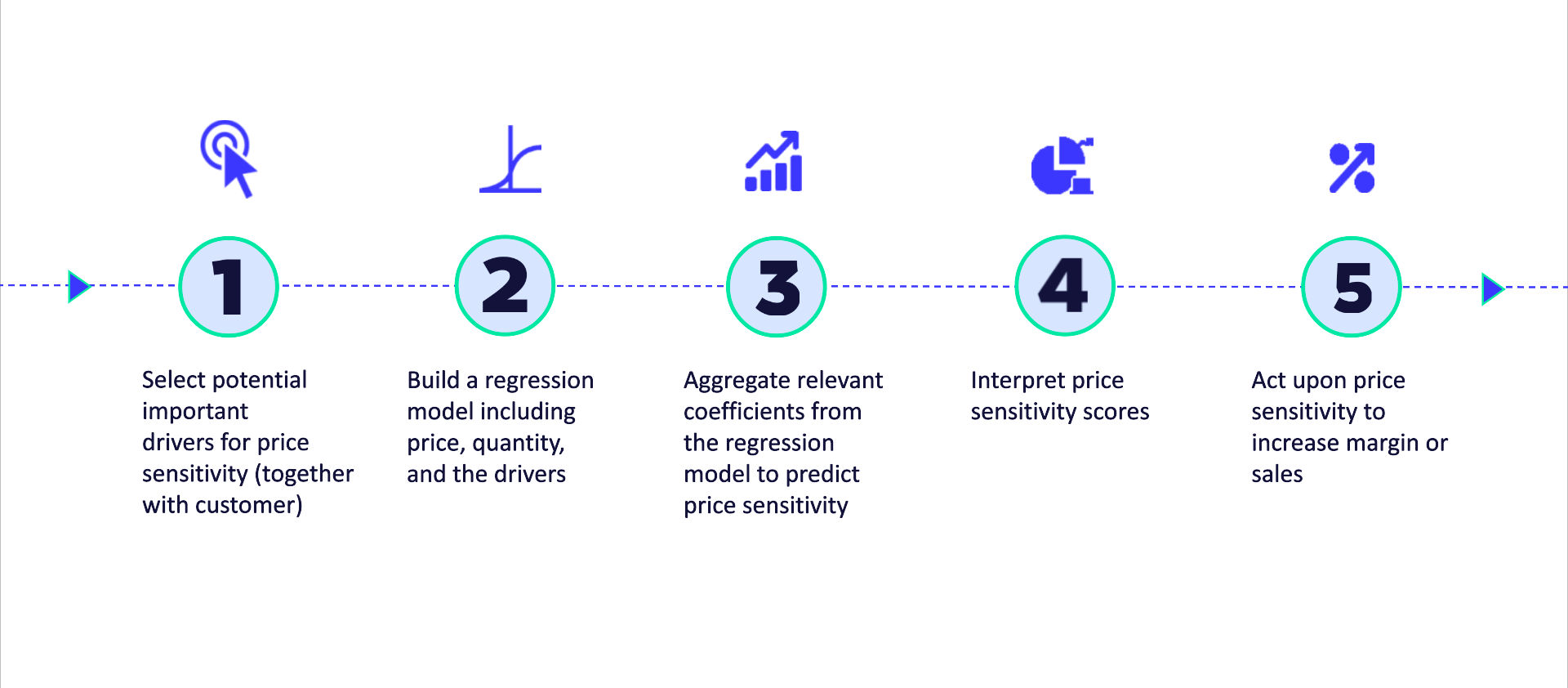
Summary: Here we look at SYMSON’s discovery of critical drivers that affect price sensitivity. The selection of these drivers was based on market research and customer surveys. They include but are not limited to:
- Brand value
- Product Lifecycle
- Price Level (as compared to competitors)
- Purchase Price changes (sudden increases or decreases in price)
- Competitive Intensity
Following this, a regression model using the relevant datasets was built to predict the price sensitivity of each product in response to a price change. Using this information, we can now group these products based on their sensitivity and apply differing price strategies to capture margin better or increase sales. For example, products in the price-sensitive group can be offered at discounts which may likely lead to higher sales. On the contrary, the products in the price-insensitive group can be priced higher. In this case, the sales may stay the same or slightly drop, but we can expect that they will be compensated for their higher prices. SYMSON is currently in the phase of testing the regression model and running experiments to determine the validity and accuracy of the model.
Assessing new key drivers for price sensitivity involves human expertise and judgement. This combined with the computational and automatization power of Machine Learning can help build a hyperlearning organisation.
Step 1: Identifying Drivers for Price Sensitivity
Engaging in market research and customer surveys helps companies gain accurate insights into customer preferences, perceptions, and purchasing decisions. At SYMSON, we discussed with a few of our clients as well as analysed data to gauge a combined perspective on the parameters that regulate their sensitivity towards purchasing a product. Of all the aspects we uncovered, here are a few drivers that could be of help to most businesses:
- Basket size:
The number of products selected in the basket size or the first product selected in the basket can impact the price sensitivity.
- Stock:
If the product is in stock or not can impact the price sensitivity. For example, whether the customer can choose an alternative if the product is not in stock can affect the price sensitivity.
- Price Change Frequency:
The number of historical price changes can impact the price sensitivity of each product.
- Brand Value:
Consumers often associate higher prices with better quality, leading to decreased sensitivity for well-established brands and vice versa for lesser-known brands.
- Product Lifecycle:
When the product is in its introductory phase, the price sensitivity may be relatively low, as customers may be willing to pay a premium for a novel or innovative product. In the growth stage, when the product gains popularity, its sales increase rapidly. The price sensitivity tends to decrease as the product becomes more established. Customers tend to be less sensitive to price changes due to increased demand and perceived value.
When the product matures reaching its peak sales and market saturation, the price sensitivity becomes typically high as the market becomes more competitive with growing alternatives to choose from. Finally, the product experiences a decline in demand as newer and better alternatives emerge. Price sensitivity can vary during this stage, but generally, customers become more price-sensitive as they may seek lower-priced alternatives or switch to newer products.
- Price Level:
This refers to whether a price is categorised as cheap or expensive. This grouping is based on customer input and sales data. A higher price may increase sensitivity. Additionally, assessing the product's price sensitivity elasticity can provide insights into the price level's impact on purchasing decisions.
- Price Change Frequency Driver:
Price Change Frequency determines the number of times a client changes the product’s price in the selected time period. High frequency suggests the price strategy follows the market dynamics closely and thus can be correlated with higher sensitivity.
- Competitive Intensity:
In a market with numerous alternatives, consumers are likelier to exhibit heightened sensitivity and seek lower-priced options. Conducting competitor analyses and staying abreast of market dynamics can help businesses gauge competitive intensity and its effect on price sensitivity.
Assessing new key drivers for price sensitivity involves human expertise and judgement. This combined with the computational and automatization power of Machine Learning can help build a hyperlearning organisation.
Step 2: Build a Regression Model including Price, Quantity, and Drivers
During a certain period, we calculated the average price, quantity, and other numerical or categorical factors for each product to build a data frame for the customer. Using this dataset, we built a regression model. This model is critical to understand the relationship between price, quantity sold, and the identified drivers of price sensitivity.
Studying these datasets enables companies to quantify the impact of each driver on price sensitivity and sales volume. Leveraging a regression analysis helped us gain a more nuanced view of how customers respond to changes in price.
Step 3: Aggregate Relevant Coefficients from the Regression Model to Predict Price Sensitivity
With this regression model in place, businesses can aggregate the relevant coefficients to predict the price sensitivity of those products. To break it down, this step uses the regression output to compute the price sensitivity scores of individual products. The equation would show a price sensitivity prediction which represents how a 1% change in price impacts the % change in quantity sold or demanded. For e.g. a price sensitivity of -4% means that a 1% rise in price results in a 4% drop in the quantity sold.
Step 4: Interpret Price Sensitivity Scores
A price sensitivity score indicates the magnitude of customer response to changes in price. Based on the estimation of the coefficients from the regression model, we give different weights to different coefficients to finally calculate the sensitivity score.
A higher sensitivity value indicates that customers are more responsive to price changes, while a lower score indicates a less significant response.
To do this, performing a test analysis on real-time price changes would provide a clear understanding of the price sensitivity of one product across multiple platforms. As our experimentation goes on, we have seen a marked difference between the price sensitivity scores over two specific platforms, one scoring significantly higher.
Step 5: Act upon Price Sensitivity to increase Margin or Sales
The final step is to translate insights from the price sensitivity analysis to increase margin. At this stage, we test it for practice to understand its performance. To do this, we segmented the product assortment into a controlled experiment group and a test group. After the sensitivity score calculation, we further divided the products within the test group into a price-sensitive group and a price-insensitive group.
Now, most of the products in the price-sensitive group are offered at discounts as it may potentially lead to higher sales due to their lower prices. On the contrary, the products in the price-insensitive group will be priced at a higher scale. The sales can stay the same or slightly drop, but we can expect the higher prices to compensate for the drop.
Likewise, by closely testing our price sensitivity scores, we can set strategies for a product group and balance sales growth/revenue generation and profit margin. We have elaborated on the experiment in the next section.
The Impact of the Price Sensitivity Assessment Approach on Business Outcomes
Summary:
Before adopting this approach, we encountered multiple issues with price elasticity and data quality. This would have led to inaccuracy in finding correct price recommendations had we continued without it. However, we see our roadblocks get solved seamlessly after adopting the price sensitivity approach. Let’s have a closer look at the outcome we witnessed and how it helped us combat the lingering issues.
- Historical Data was more organised and ready to be used for the Optimisation
With the implementation of the price sensitivity assessment approach, SYMSON experienced a remarkable transformation in historical data management. Earlier, we found our historical datasets in an unorganised manner which made it difficult to use them for price optimisation. Now, with the price sensitivity assessment approach, we could include more price factors and use them to make more accurate price suggestions.
- Availability of Competitive Pricing Data
The 5-step approach not only considered internal data but also helped equip us with accurate datasets about the price sensitivity of competitor products. Further, it provides a direction to either set a discount or mark up. In the case that your competitor is not available, this approach can offer you a sense of price optimisation.
- Increased Accuracy in Finding an Optimal Balance between Profit Optimisation and Risk Management
As we researched (combined customer and data perspectives) to find additional drivers for sensitivity, we can understand the demand level, of the products in question, with more precision. Of course, price isn’t a lone factor that makes demand fluctuate significantly. Hence, the newly researched drivers are proving to ensure accurate demand trends, customer behaviour and enhanced demand predictions. That said, it will help us gauge the optimal price recommendations for increased revenue and profit growth.
This method allows for risk management as well. For example, very high discounts or markups can affect sales too heavily. By setting conservative discounts and markups the price sensitivity predictions can be tested and measured in impact.
- Sales Teams gained Confidence in the Outcomes to discuss them with Customers.
Incorporating this clear and logical approach into the pricing strategy that included several practical drivers, we saw our sales team gain confidence in the sensitivity outcomes. Previously, the word wasn’t presented to the customers as we lacked clarity in the datasets and elasticity predictions.
To get a buy-in from the sales team, we interviewed them regarding the factors to include in the model. Below is a heatmap, that we created after making the model, to make the outcomes understandable and explainable. It shows how each factor affects the sensitivity per product. Furthermore, it proved to be more extensive and accessible than a price elasticity approach.

5 Benefits of Embracing Price Sensitivity for Price Optimisation
As markets transform into dynamic spaces, businesses need to refine their price optimisation tactics to keep pace. Identifying new parameters that regulate purchase decisions and understanding consumer behaviour towards a specific product category help maintain margin growth and brand identity in the long run. While there are several other profitable benefits of implementing price sensitivity for price optimisation, we present the top five.
1. Enable Demand Forecasting with Precision
Conducting new driver-research exercises helps ensure better accuracy in identifying product demand. With additional practical parameters in place, the final price recommendation outcome would be precise. Furthermore, it creates future demand predictions that prepare organisations to set strategies for correct pricing suggestions.
2. Optimal Pricing Strategies for Sales and Margin Growth
As price sensitivity includes several new drivers, the pricing strategy will provide recommendations that are the closest to reality. This approach refines the price optimisation process ensuring accurate suggestions that are accurately feasible for customers and also provide optimal profit for the company.
With proper identification of the nature of the products and demarcation into their feature groups, businesses can not only assign discount and markup strategies but also combine additional strategies with respect to the market and competitive scenario.
3. Capitalise on Price-insensitive Products to improve Margins
With the clarity of the product assortment’s price sensitivity, companies can identify items with unresponsive demand during price changes. This opens up the ground for raising prices to a certain level that not only increases profit margins but also is within the range of customer’s willingness to pay. The method frees up the pressure to increase sales volume while also boosting profitability.
4. Strengthen Customer Loyalty with Personalised Pricing
Businesses offering value-based pricing and personalised promotions that align with buyer preferences make customers perceive it positively. Moreover, consistency in this approach leads to brand loyalty and eventually, advocacy. Lasting customer relationships also save businesses the costs of acquiring new customers.
5. Stay ahead of the Competition with regular Pricing Analysis
With comprehensive pricing strategies in place, brands can beat competitors in dynamic markets. Continuous analysis of customer behaviour and competitors' prices by intelligent pricing tools helps set new prices with respect to the minimum margin and cap adjustment set in business rules.
Implementing price sensitivity assessment in smart price optimisation tools like SYMSON paves the way for a successful pricing journey, positioning businesses to thrive in ever-evolving market landscapes.
HyperlearningTM: The Future of AI Adoption
In the article Building a More Intelligent Enterprise, Paul Schoemaker shows us a visualisation of the differing areas of strengths for humans vs computers and a new category of areas where humans and computers are stronger together. He explains that these categories are grouped based on the familiarity of problems and the available density of data.
In the price sensitivity approach, human expertise excels in assessing price sensitivity drivers and prioritizing them according to their importance. Computers are outperforming them in finding patterns, building the algorithms and computing all scores. However, in the end, computers and humans must work together to assess which products are practically sensitive.
Business managers must evaluate the Machine Learning outcomes so that the model can continuously improve and enhance the price sensitivity scores with precision. Moreover, this is a continuous process where the business experts learn from data insights, and the model is updated. This is what we call HyperlearningTM where both worlds (business and AI) operate with fine cooperation and an awareness of each strength.

Combining Machine Learning with Human Expertise
Price optimisation serves as a prime example of the potential impact of combining human expertise and machine learning. Price sensitivity analysis, conducted through regression models and machine learning, allows organisations to understand customer behaviour patterns, facilitating more accurate price-setting decisions. By integrating historical data on customer preferences, buying behaviours, and market trends, businesses can optimise prices to maximise profitability and customer satisfaction.
AI-powered pricing algorithms can rapidly analyse vast amounts of data, including competitor pricing, customer demographics, and market trends, to adjust prices in real-time dynamically. By combining AI's data-driven decision-making capabilities with human expertise in understanding consumer psychology and market dynamics, businesses can strike a delicate balance between competitive pricing and maintaining profit margins.
In other words, combining human expertise and machine learning is the cornerstone of building a more intelligent enterprise.
Conclusion
It is a well-known phenomenon that in many companies the data of recent years is insufficient and inaccurate to measure price elasticity and thus predict prices. Machine learning's ability to handle high-dimensional data, uncover complex patterns, enhances accuracy and provides a competitive advantage.
This article provides insight into this approach in which more variables and limited recent data can be included to map price sensitivity. The human judgment adds a unique perspective and understanding of customer behaviour and market dynamics. The combination of both these elements creates a more intelligent enterprise capable of making informed decisions, adapting pricing strategies, and maintaining a competitive edge.
This method is based on a close collaboration between the human expertise in the company and machine learning technology. This leads to greater adoption by sales, better risk management of price adjustments and high accuracy.
The fusion of human expertise and machine learning in price optimisation emerges as a pivotal factor for businesses seeking sustainable growth and success in the data-driven business landscape.
Do you want a free demo to try how SYMSON can help your business with margin improvement or pricing management? Do you want to learn more? Schedule a call with a consultant and book a 20 minute brainstorm session!